SLM series: NTT DATA - Cost-effective solutions for real-time industrial AI
This is a guest post for the Computer Weekly Developer Network written by Shahid Ahmed in his role as EVP for Edge Services at NTT Data.
Part of the wider NTT conglomerate family, NTT Data (stylised as NTT DATA) is known for its client-first IT consulting and modernisation services that gravitate around the most widely used (and some of the most esoteric and emerging) areas of software engineering and data science.
The company is also known for what it calls its discovery-as-a-service approach which uses “blended teams” to analyse enterprise IT stack deployments with discoveries that yield evidence-based recommendations.
Ahmed writes in full as follows…
Large Language models or LLMs, such as GPT, Anthropic and Gemini have dominated the market.
The capabilities of these models, powered by vast datasets, can produce realistic images and videos, handle intricate queries and produce multi-modal human-like language.
However, for industries and enterprises that require real-time decision-making, cost efficiency and localised processing, LLMs often present significant cost challenges and don’t quite fit the bill.
Instead, small or lightweight language models offer a more practical alternative for enterprises using AI to boost productivity. Think robotic arms and other factory operational technologies (OT). This is called physical AI.
The limits of LLM implementation
LLMs excel at solving complex queries and generating sophisticated responses and reasoning, but their time-series-based operational capabilities can be [limitingly] prohibitive. They demand substantial computational resources, including memory and processing power, which raise implementation and maintenance expenses.
This is evident by the recent paradigm shift in AI as a result of the DeepSeek R1 launch. Furthermore, their reliance on cloud-based processing introduces latency and connectivity issues, which can hinder performance in environments where speed and reliability are critical.
Such reliance can lead to operational disruptions in areas with limited or unreliable connectivity. For example, consider a factory floor where AI helps monitor and operate machinery. In this environment, decisions must be made instantly to ensure safety and maintain operational efficiency. Delays caused by low latency can disrupt operations, potentially leading to costly downtimes and security hazards. The need to transmit data to the cloud also creates vulnerabilities around sensitive or proprietary data.
These limitations highlight why LLMs may not always be suitable for enterprise needs.
Enter SLMs
SLMs are designed to bring the benefits of AI to enterprises without the limits of their larger counterparts, offering distinct advantages:
- Low Latency: Unlike LLMs, SLMs can run locally on edge devices, eliminating the latency associated with cloud-based processing, which enables real- or near-real-time decision-making.
- Cost-Effectiveness: SLMs require fewer computational resources for pre- and post-training and deployment, making them a more budget-friendly option for enterprises.
- Task-Specific Optimisation: SLMs can be fine-tuned for specific tasks or domains, making them more efficient and enabling higher accuracy in applications, such as predictive maintenance, anomaly detection and real-time analytics.
Data pipelines for SLMs
Although SLMs (which are generally mathematical-based) are an effective tool for enterprises, their full potential cannot be achieved until data is gathered, managed and transformed.
This means creating systems and tools, such as edge AI, that identify and gather high-quality data which is essential to particular tasks, such as aggregating all of the sensor readings in a manufacturing environment. This drives the significant trend of IT/OT convergence – that combines IT systems, which manage data and communications, with OT systems, which control physical devices, equipment and industrial operations.
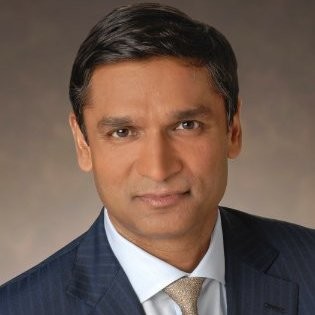
NTT DATA’s Ahmed: SLMs are designed to bring the benefits of AI to enterprises without the limits of their larger counterparts, offering distinct advantages:
Edge AI systems are paving the way for a new era of digital transformation.
By continuously collecting data from an expanded array of sensors, machinery, cameras and applications, manufacturers can enhance processes like maintenance scheduling, inventory and safety.
Another key strength of edge AI lies in its ability to transform vast amounts of unstructured data into a unified data plane, enabling the development of impactful AI use cases in operations that were previously unable to leverage such technology. The SLMs can then utilise this data to learn and start making inferences in real-time.
The small-scale AI model can be trained in a manufacturing setting with information about what constitutes good and bad product units. Using that data, the model can accurately identify a wider range of potential issues, alert staff, suggest repair procedures and even estimate repair costs before equipment failure.
Simplifying AI with managed services
As demand for AI grows, managed services play a crucial role in simplifying the deployment and maintenance of SLMs. These service providers equip enterprises with tools, expertise and ongoing support, enabling even small teams to implement and sustain effective AI. They also allow businesses to focus on innovation and growth with the assurance that their AI systems remain optimised.
From the factory floor to mining operations to airport baggage handling systems to the hospital corridor, SLM AI solutions are revolutionising industries by addressing real-world challenges. Their tailored, cost-effective solutions bring AI to the forefront where it matters most, delivering tangible benefits for workers and organisations alike.
By embracing SLMs, enterprises can advance in efficiency, safety and operational excellence – ushering in a new era of industrial transformation.