SLM series - Iterate.ai : Strategic sweet spots for sustainable savviness
This is a guest post by Shomron Jacob, head of applied machine learning & platform at Iterate.ai, whose AI innovation ecosystem enables enterprises to build production-ready applications.
Previously, he worked at Apple on various emerging technology projects that included the Mac operating system and the first iPhone.
As tech giants wage an LLM arms race – committing billions to build increasingly massive AI systems – they’re careening toward a financial cliff edge. This high-stakes game of chicken raises the question: who will be first to admit that unlimited CapEx spending on AI is fundamentally unsustainable?
Businesses across every sector have plunged headfirst into LLM investments, with many now stretching resources dangerously thin. With the latest GPUs now commanding tens of thousands of dollars and cloud bills harder and harder to stomach, the economics have become unsustainable. The time to reassess longer-term AI strategies is now.
Enter SLMs – small language models that deliver comparable AI functionality and benefits as their larger counterparts while requiring just a fraction of the computational resources. These lightweight alternatives are precision instruments, not sledgehammers, that offer businesses a practical and more private, escape from the LLM spending trap.
SLM advantages: faster training, reduced environmental impact, sustainable costs
Whereas LLMs blast away like industrial firehose – drowning problems in up to a trillion parameters – SLMs deliver outputs using under 10 billion parameters.
The efficiency gains become even more dramatic with fine-tuned SLMs using retrieval-augmented generation (RAG), which can achieve great results with a mere 70,000 parameters. While SLMs excel at targeted use cases rather than general-purpose applications, companies that strategically deploy both technologies gain a competitive advantage. Like choosing between power tools and hand tools, organisations can select the right approach for each task, maximising efficiency without sacrificing performance.
The hardware economics tell a compelling story.
While LLMs demand expensive racks of specialised GPUs, SLMs can run efficiently on NPUs, conventional CPUs, or minimal GPU configurations. This translates directly to the bottom line i.e. training times shrink from months to weeks, dramatically accelerating business agility across the entire AI lifecycle. More importantly, SLMs’ focused design often delivers superior performance within their specific domains, outpacing what general-purpose LLMs achieve.
More precision enables AI engineering teams to address business requirements with more speed and accuracy, slashing time-to-market while delivering more polished, purpose-built AI solutions.
The environmental case for SLMs is equally compelling. As LLMs face mounting criticism for their staggering ecological footprint – with damning headlines about their electricity consumption rivaling small nations, depleting water reserves and generating massive carbon emissions – SLMs offer businesses a responsible alternative.
A competitive edge
While cloud-based options exist, self-hosted SLMs deliver a decisive advantage in both security and competitive differentiation. Businesses using public cloud LLMs face a trade-off: feeding their proprietary data into systems that learn from it…and then deliver identical capabilities to competitors paying for the same service.
With private SLMs, companies maintain complete ownership of their AI ecosystem – turning their unique data assets into exclusive competitive advantages that rivals can’t replicate. The security benefits are also big, as sensitive information never leaves corporate infrastructure, substantially reducing compliance risks and eliminating thorny data licensing issues that plague public LLM deployments.
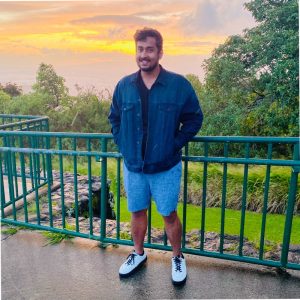
Shomron Jacob is the head of applied machine learning & platform at Iterate.ai
The benefits extend across virtually every industry vertical, because virtually every industry vertical has use cases requiring rapid, intelligent access to their organisation’s proprietary data.
Consider customer service platforms that instantly surface relevant information from multiple databases, delivering personalised solutions in seconds rather than minutes. Or retail loyalty programs that dynamically generate tailored offers based on individual shopping patterns, driving dramatic increases in conversion rates.
In healthcare, SLMs can process thousands of patient records and medical documents to accelerate diagnostics and treatment planning, while financial services firms deploy them to analyse complex contractual language and regulatory requirements without exposing sensitive client information. Across all these scenarios, SLMs flex computational muscle to revolutionise core business processes while maintaining the agility and cost-efficiency that cumbersome LLMs simply cannot match.
Right-sizing your AI strategy
The future of business AI isn’t about choosing between SLMs and LLMs – it’s about strategic deployment of both.
Like championship basketball coaches who adjust lineups based on game situations, successful organisations will develop a tactical approach to AI implementation: deploying nimble SLMs when speed and efficiency are paramount and reserving resource-intensive LLMs for specialised applications requiring their broader capabilities.
Many teams are finding success by starting with focused SLM deployments to prove concept and generate quick wins, then selectively scaling to larger models as specific use cases demonstrate clear ROI.
As the AI landscape continues its rapid evolution, this flexible, right-sized approach to language models will separate industry leaders from the pack – delivering sustainable innovation while avoiding the financial pitfalls of over-engineered solutions.