SLM series - IBM: Why smaller AI models unlock value at the edge
This is a guest post for the Computer Weekly Developer Network written by Dr Juan Bernabe-Moreno in his capacity as director of IBM Research Europe for the UK & Ireland.
Bernabe-Moreno writes in full as follows…
The rise of generative AI has put the term ‘large language models (LLMs)’ firmly into mainstream vocabulary. From automating content creation to extracting insights and chatting with customers, the possibilities of these models seem endless. However, they also come with big challenges.
They are costly to develop, require vast amounts of energy and demand powerful infrastructure to run effectively. There are also concerns about data privacy, especially when models are hosted on the cloud and trained on unstructured, publicly available data.
Enter Small Language Models (SLMs) – a new generation of models that promises to address these challenges while unlocking more business value from AI.
The power of small
SLMs are a type of Small Foundation Model (SFM), focused on language. While traditional LLMs can contain hundreds of billions of parameters, SLMs operate with only a fraction of that—typically between one to three billion parameters.
This means they require far less computational power, energy and financial investment to develop and use. For enterprises, this translates to a big reduction in costs, making AI tools accessible to businesses of all sizes, as well as individuals.
Despite their modest size, SLMs, such as IBM Granite models, have been shown to perform just as well – or better – than LLMs based on standard industry benchmarks.
Smaller models on the edge
One area where SLMs, as well as generic SFMs trained on different types of data, truly shine is in edge computing—where data is processed locally on devices rather than being sent to the cloud for analysis.
Edge devices such as smartphones and smart sensors on factory floors have limited computing power and storage capacity, but they generate massive amounts of data in real-time. SLMs and SFMs allow businesses to process this data on the spot, unlocking valuable insights without relying on centralised infrastructure.
Manufacturing makes it
Traditional factory operations generate vast amounts of real-time data, but only a fraction of it is currently being used. With SFMs deployed at the edge, businesses can begin to unlock the value hidden within this untapped data.
SFMs used at the edge can also improve industry applications of computer vision technology. Currently, computer vision algorithms in automobiles can stop a car if they detect an object like a ball within a certain proximity of the vehicle. As SLMs and SFMs become more sophisticated, they learn from experience, detecting patterns and making predictions.
For example, if a car can detect and recognise a soccer ball, it might be able to predict that a child will come out to pick up the ball a few seconds later and react accordingly.
In a related example, IBM’s Supply Chain Engineering team developed a system that uses AI on the edge to inspect the quality of products in real-time. Tasks that used to take engineers several minutes – like checking if a server has all the screws in place – can now be done in under a minute, thanks to devices powered by small AI models. This means faster production times and fewer human errors.
Privacy & security
As businesses turn to AI for everyday tasks, data privacy and security remain top concerns, particularly in regulated industries like healthcare and finance. One advantage of smaller models is that they can operate entirely on local devices, removing the need to transmit sensitive data to the cloud.
This means businesses can maintain greater control over their data, offering enhanced privacy protections compared to cloud-based models. With data staying on-device, there is also less risk of data breaches or unauthorised access.
Expanding access to AI
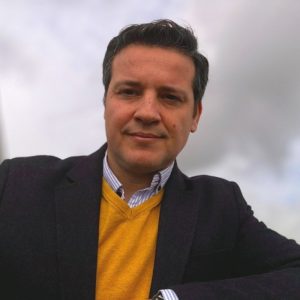
IBM’s Bernabe-Moreno: High accuracy at a fraction of the cost, SLMs & SFMs create new opportunities for businesses & individuals alike.
One of the most exciting aspects of SLMs is their ability to bring powerful AI capabilities to a far broader audience. With their reduced resource demands, SLMs can be deployed by businesses without massive infrastructure investments and individuals can even run these models on their smartphones.
SLMs can also work offline, allowing them to be useful in areas without internet access. This democratisation of AI allows smaller businesses, rural communities and people in developing regions to benefit from AI tools previously reserved for the most well-funded organisations.
Consider, for example, a farmer in a rural region who spots signs of disease on a crop. With a small vision model running on their smartphone, they can snap a photo and immediately receive a detailed analysis and treatment suggestions – no Internet required.
Looking ahead
While still in the early stages, the potential of smaller models is clear. These compact yet highly efficient models represent the future of AI, particularly where resources are limited and data needs to be processed in real-time. By providing high accuracy at a fraction of the cost of larger models, SLMs and SFMs create new opportunities for businesses and individuals alike, especially in edge computing applications.
With these powerful, small models, we are not just changing how AI works, but who gets to use it and where.