SLM series - IBM: Open source base models empower data-infused specialised models
This is a guest post for the Computer Weekly Developer Network written by Raj Datta, vice president, software and AI partnerships at IBM.
Datta writes as follows…
In 2025, software companies wishing to quickly advance their AI solutions are turning to small language models (SLMs).
SLM models are enticing as they contain smaller datasets which are faster to train and deploy, requiring less computational power and memory. These are designed for specific enterprise tasks – and are noticeably outperforming or matching similarly sized models.
The market for SLMs is expected to grow a steady 15% over the next five years, likely because they can be implemented with limited resources.
Buttery biscuit base (model)
SLMs can be useful to companies looking for targeted quick wins – and are even more powerful when open source. We at IBM believe open source base models empower enterprises to create the best data-infused specialised models. This is why we open sourced our Granite family of customisable SLMs.
These SLMs are trained on filtered and transparent datasets, achieving high-cost savings.
By combining a small Granite model with enterprise data, tasks can be completed at a fraction of the cost when compared to larger models. That’s why SLMs are preferable for the masses as they use far fewer parameters and can be built from scratch or adapted from LLMs. Due to the smaller size of the models, they can be hosted in a datacentre rather than the cloud. Further, because these can be trained on curated enterprise datasets, SLMs are more easily filtered for objectionable content; governance, privacy, risk and bias mitigation is more important than ever.
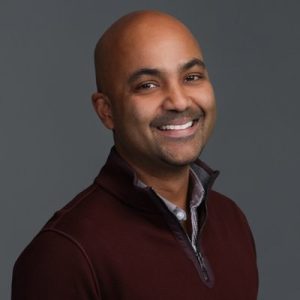
IBM’s Datta: IBM believes open source base models empower enterprises to create the best data-infused specialised models.
Agentic AI
We predict SLMs will be very useful in the advancement of AI agents.
SLMs feature support for practical agentic capabilities and with these models, advanced reasoning and specific function calling can be achieved. This is essential to ensure agents can connect with external APIs, reassess plans of action and even self-correct. Enterprises can fine-tune SLMs to power customer service chatbots that are capable of responding rapidly in real-time. They can also serve as the foundation for agentic AI chatbots – that can complete tasks on command.
Conversely to the SLM small dataset nature, large language models (LLMs) are trained with massive datasets.
Due to the larger datasets, the data can take months to train and for humans to fine-tune. LLMs are not cheap, and these models involve significant development expenses that may run into several million dollars, which can be a major financial burden for most software companies and startups.
Looking ahead, some companies may prefer a combination of LLMs and SLMs. Using bigger models first can be effective to address the most challenging business problems. Then, shifting to smaller models can replicate the findings at a lower cost and with decreased latency.