SLM series: ABBYY - A strategic recalibration of the tech arsenal
This is a guest post for the Computer Weekly Developer Network (CWDN) written by Maxime Vermeir in his role as senior director of AI strategy at ABBYY.
Vermeir writes in full as follows…
On the question of whether SLMs and LLMs be combined and used in union and in concert — LLMs and SLMs each have their own strengths and weaknesses, so naturally it follows that combining them can balance the risks of both.
LLMs, trained on vast amounts of data, possess a broad understanding of language and the world. This makes them incredibly versatile, but they can also be slow and resource-intensive and can take up to 50 times longer to process information compared to smaller models.
Parameter perimeters
SLMs are purpose-built to be more efficient, with parameter counts ranging from 1 to 10 million. This streamlined design makes SLMs faster and more efficient to train and deploy, making them ideal for tasks that require speed and precision. Combining the two meets different needs for a business.
LLMs are great for tasks that need a deep understanding of context, while SLMs are better for specific, focused tasks. SLMs are often used as part of larger systems to handle specialised functions more efficiently. A variety of specialised SLMs can often outperform a single general-purpose LLM, especially when businesses are prioritising speed, cost and privacy.
However, there are situations where LLMs could be the better choice due to their broader reasoning and deep contextual understanding.
LLM models can answer a broad range of questions and summarise lots of types of information and even come up with creative content with remarkable accuracy. General-purpose, open-source LLMs like ChatGPT and Gemini can also be tailored and pre-trained for specialised business purposes.
A strategic recalibration
It’s important to remember that LLMs, while transformative, represent just one of many tools in our collective arsenal as technologists (and, in my case as a representative of ABBYY).
I view the shift from LLMs to SLMs not as a groundbreaking discovery, but rather as a strategic recalibration – an opportunity to be more intentional and focused with AI applications.
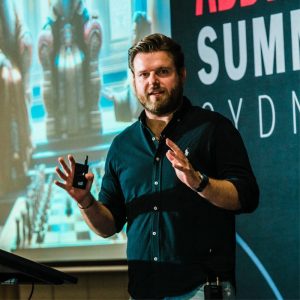
ABBYY’s Vermeir: LLMs, while transformative, represent just one of many tools in our collective arsenal as technologists.
The fact that SLMs are generally faster, more affordable and easier to train makes them ideal for specific and targeted tasks like, for example, intelligent document processing (IDP). SLMs learn from a smaller, more focused set of data that’s directly relevant to the tasks they’re designed for. This makes them quicker to train, faster to operate and more environmentally friendly.
Every organisation has unique needs which SLMs can be customised to meet, for everything from managing legal forms to scheduling healthcare appointments.
These models can be trained on a business’s specific data to become experts in their specific field. There’s no ‘one size fits all’ approach.”
Environmental sustainability
With organisations increasingly striving to meet tightening ESG standards, it’s important to prioritise the sustainability implications of training AI. We’ve noticed a pivot towards SLMs in response.
Generative AI consumes considerably more energy compared to other types.
A study from Indiana and Jackson State University found the carbon footprint of GPT-3 trained by different computing devices are the equivalent of a roundtrip flight from San Francisco to New York. SLM models have lower energy consumption, due to the more streamlined data necessary for them to run. SLMs require less data, meaning their training and inference also have lower carbon emissions. Employing targeted systems allows businesses to reduce environmental impacts while effectively solving specialised problems.
SLMs are designed to be used by enterprises for specialised tasks that require precision and consistency. This makes them ideal for specific tasks such as Intelligent Document Processing (IDP), where the extraction, organisation and processing of data from documents is automated.
Getting smart with IDP
IDP works with any document type and format to process content in documents like a human.
SLMs’ ability to efficiently process large volumes of documents with minimal resources makes them a great fit for automating and streamlining document-related workflows in businesses.
Another popular use for SLMs is in applications like chatbots, which provide instant customer service. These models can quickly respond to inquiries, handle common issues and guide customers through processes.